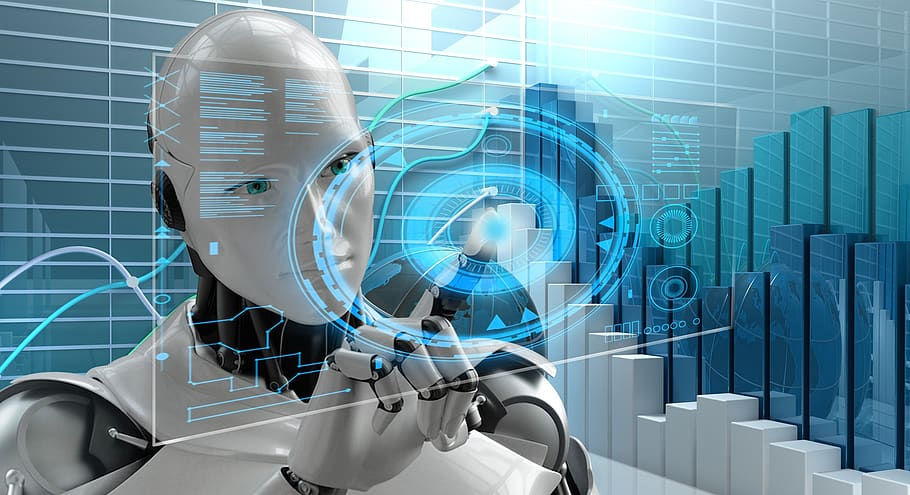
Machine learning has transformed numerous industries by enabling computers to learn from data and make intelligent decisions. As we look towards the future of this field, one particular area gaining significant attention is unsupervised learning. In this article, we will explore the potential of unsupervised learning and its role in shaping the future of machine learning, revolutionizing how computers learn and discover patterns without explicit guidance.
Understanding Unsupervised Learning: Unsupervised learning is a branch of machine learning where algorithms are trained on unlabeled data. Unlike supervised learning, which relies on labeled examples to make predictions, unsupervised learning seeks to uncover patterns, structures, and relationships within data without any predefined target variable. It allows machines to learn from the inherent properties of the data itself.
The Power of Unsupervised Learning: Unsupervised learning holds immense promise for the future of machine learning due to its ability to uncover hidden insights and discover novel knowledge. By exploring vast amounts of unlabeled data, unsupervised learning algorithms can identify patterns and structures that may not be apparent to humans. This makes it a valuable tool for data exploration, feature engineering, and anomaly detection.
Clustering: Grouping Similar Patterns: Clustering is a common unsupervised learning technique that involves grouping similar data points together based on their inherent similarities. By employing algorithms such as k-means, hierarchical clustering, or density-based clustering, machines can automatically identify clusters within a dataset. This has applications in customer segmentation, recommendation systems, and anomaly detection.
Dimensionality Reduction: Simplifying Complex Data: Unsupervised learning techniques like Principal Component Analysis (PCA) and t-SNE (t-Distributed Stochastic Neighbor Embedding) help simplify high-dimensional data by reducing its dimensionality while retaining critical information. By transforming complex data into a lower-dimensional space, these techniques facilitate data visualization, pattern recognition, and feature extraction.
Generative Models: Creating New Data: Generative models, such as Variational Autoencoders (VAEs) and Generative Adversarial Networks (GANs), are at the forefront of unsupervised learning advancements. These models learn the underlying data distribution and can generate new, realistic samples from that distribution. Generative models have applications in image synthesis, data augmentation, and content generation.
Challenges and Future Directions: While unsupervised learning has made remarkable progress, challenges remain. Evaluation metrics and assessing the quality of unsupervised models can be subjective and complex. Additionally, scalability and interpretability of unsupervised techniques are areas of active research. However, advancements in deep learning, reinforcement learning, and hybrid approaches show promise in overcoming these challenges.
Real-World Applications: Unsupervised learning is already making a significant impact across various domains. In finance, it helps detect fraudulent transactions by identifying unusual patterns. In healthcare, it aids in patient stratification and drug discovery. In cybersecurity, it assists in identifying network intrusions. The potential applications are vast and extend to recommendation systems, natural language processing, and more.
Unsupervised learning is poised to shape the future of machine learning, providing powerful tools for data exploration, pattern recognition, and knowledge discovery. As algorithms continue to evolve and researchers delve deeper into unsupervised techniques, we can expect significant advancements in areas such as clustering, dimensionality reduction, and generative models. Embracing the potential of unsupervised learning opens doors to new insights and applications, propelling machine learning into exciting and uncharted territories.
Remember, optimizing your article for search engines involves more than just incorporating keywords. It's essential to provide valuable and engaging content for readers while ensuring proper keyword placement to improve visibility and reach.
Comentarios